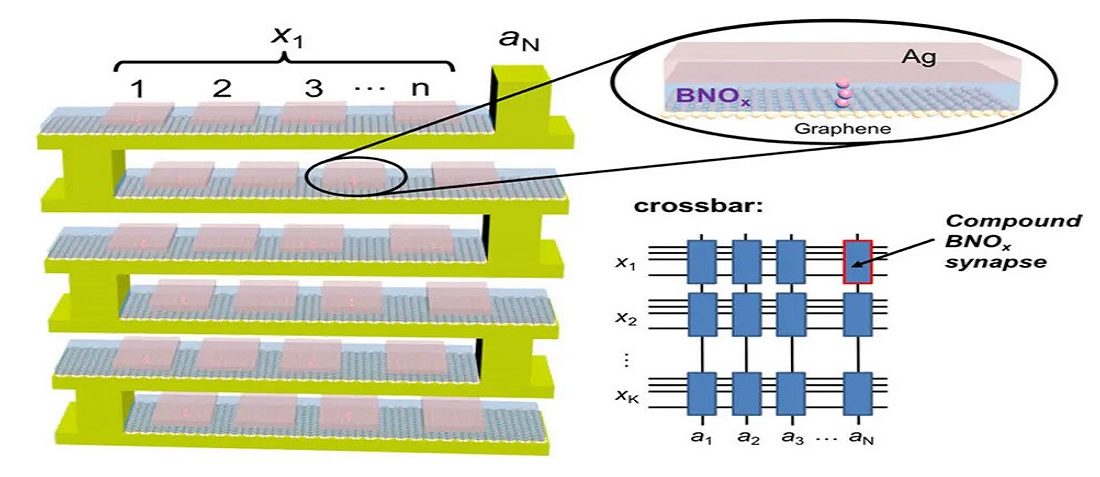
New Memristor Boosts Accuracy and Efficiency For Neural Networks on an Atomic Scale
- Posted by doEEEt Media Group
- On June 23, 2023
- 0
A new parallel processing approach for building resistance-based artificial synapses promises to ramp up efficiency and accuracy by making memristors as thin as a single atom.
From the Journal: Journal of Applied Physics.
WASHINGTON, D.C., October 16, 2018 — Just like their biological counterparts, hardware that mimics the brain’s neural circuitry requires building blocks that can adjust how they synapse, with some connections strengthening at the expense of others. One such approach, memristors, uses current resistance to store this information. New work looks to overcome reliability issues in these devices by scaling memristors to the atomic level.
A group of researchers demonstrated a new type of compound synapse that can achieve synaptic weight programming and conduct vector-matrix multiplication with significant advances over the current state of the art. Publishing its work in the Journal of Applied Physics, from AIP Publishing, the group’s compound synapse is constructed with atomically thin boron nitride memristors running in parallel to ensure efficiency and accuracy.
The article appears in a special topic section of the journal devoted to “New Physics and Materials for Neuromorphic Computation,” which highlights new developments in physics and materials science research that hold promise for developing the very large-scale, integrated “neuromorphic” systems of tomorrow that will carry computation beyond the limitations of current semiconductors today.
“There’s a lot of interest in using new materials for memristors,” said Ivan Sanchez Esqueda, an author of the paper. “We’re showing that filamentary devices can work well for neuromorphic computing applications when constructed in new clever ways.”
Current memristor technology suffers from a wide variation in how signals are stored and read across devices, both for different types of memristors and different runs of the same memristor. To overcome this, the researchers ran several memristors in parallel. The combined output can achieve accuracies up to five times those of conventional devices, an advantage that compounds as devices become more complex.
The choice to go to the subnanometer level, Sanchez said, was born out of an interest to keep these parallel memristors energy-efficient. An array of the group’s memristors were 10,000 times more energy-efficient than currently available.
“It turns out if you start to increase the number of devices in parallel, you can see large benefits in accuracy while still conserving power,” Sanchez said. Sanchez said the team next looks to further showcase the potential of the compound synapses by demonstrating their use in completing increasingly complex tasks, such as image and pattern recognition.
Source: AIP publishing highlights
featured image: A conceptual schematic of the 3D implementation of compound synapses constructed with boron nitride oxide (BNOx) binary memristors and the crossbar array with compound BNOx synapses for neuromorphic computing applications. CREDIT: Ivan Sanchez Esqueda
- Miniature RF Connectors for high-performance testing - April 24, 2025
- Space-Grade components available for immediate delivery - April 10, 2025
- Managing EEE components for LEO and lower cost space missions - December 17, 2024
0 comments on New Memristor Boosts Accuracy and Efficiency For Neural Networks on an Atomic Scale